报告题目:Distributed Learning in e-health
报 告 人:Kuan Zhang, Assistant Professor, University of Nebraska-Lincoln
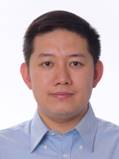
邀请人:李雪莲 副教授
报告时间:2022年11月27日(周日)9:00-10:30
腾讯会议ID:486-366-056
报告人简介:Dr. Kuan Zhang is an Assistant Professor with the Department of Electrical and Computer Engineering, University of Nebraska–Lincoln, USA. He received the Ph.D. degree in electrical and computer engineering from the University of Waterloo, Canada, in 2016. He was a postdoctoral fellow at the Department of Electrical and Computer Engineering, University of Waterloo from 2016-2017. He has published over 100 papers in journals and conferences. His research interests include cyber security, big data, and cloud/edge computing. Dr. Zhang received the Outstanding Ph.D Thesis Award of IEEE Technical Committee on Scalable Computing (TCSC) Award for Excellence in 2017. He was a recipient of the Best Paper Award at IEEE WCNC 2013, Securecomm 2016, and ICC 2020.He is associate editor of IEEE Transactions on Wireless Communication, IEEE Communications Surveys & Tutorials, IEEE Internet-of-Things Journal, Peer-to-Peer Network and Applications.
报告摘要:E-health allows smart devices and medical institutions to collaboratively analyze patients’ data, which is trained by Artificial Intelligence (AI) to help doctors make diagnosis. By allowing multiple devices to train models collaboratively, federated learning is a promising solution to address the communication and privacy issues in e-health. However, applying federated learning in e-health faces many challenges. For example, medical data is both horizontally and vertically partitioned. Since single Horizontal Federated Learning (HFL) or Vertical Federated Learning (VFL) techniques cannot deal with both types of data partitioning, directly applying them may consume excessive communication costs due to transmitting a part of raw data when requiring high modeling accuracy. In this work, we present a thorough study on an effective integration of HFL and VFL, to achieve communication efficiency and overcome the above limitations when data is both horizontally and vertically partitioned. Specifically, we propose a hybrid federated learning framework with one intermediate result exchange and two aggregation phases. Based on this framework, we develop a Hybrid Stochastic Gradient Descent (HSGD) algorithm to train models. Then, we theoretically analyze the convergence upper bound of the proposed algorithm. Using the convergence results, we design adaptive strategies to adjust the training parameters and shrink the size of transmitted data. Experimental results validate that the proposed HSGD algorithm can achieve the desired accuracy while reducing the communication cost, and they also verify the effectiveness of the adaptive strategies.