报告题目:Learning a Class-Aware Sample Weighting Mapping for Robust Deep Learning
报告人: 束俊 博士 西安交通大学
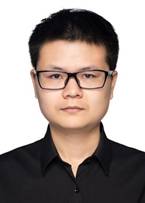
邀请人:贾西西
报告时间:2022年4月8日(周五)10:00——11:30
腾讯会议:607 453 608
报告摘要:
Modern deep neural networks (DNNs) can easily overfit to biased training data containing corrupted labels or class imbalance. Sample re-weighting methods are popularly used to alleviate this data bias issue. Most current methods, however, require to manually pre-specify the weighting schemes as well as their additional hyper-parameters relying on the characteristics of the investigated problem and training data. This makes them fairly hard to be generally applied in practical scenarios, due to their significant complexities and inter-class variations of data bias situations. In this report, we will show our proposed meta learning method for addressing this issue, and we achieve proper weighting schemes in various data bias cases, like the class imbalance, feature-independent and dependent label noise scenarios, and more complicated bias scenarios beyond conventional cases.
报告人简介:
Jun Shu received the B.E. degree from Xi'an Jiaotong University, Xi'an, China, in 2016, where he is currently pursuing the Ph.D degree, under the tuition of Prof. Deyu Meng and Prof. Zongben Xu. His current research interests include machine learning and computer vision, especially on meta learning, robust deep learning and AutoML.He is/was a PC member of top-conferences such as ICML/NeurIPS/ICLR/CVPR/ECCV/IJCAI, and a reviewer of journals like the IEEE TPAMI, SCIENCE CHINA Information Sciences, etc.