报告题目:Model Checking in Large-Scale Data Set via Structure-Adaptive-Sampling
报告人:韩艺欣 博士生 南开大学
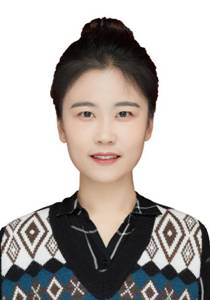
邀请人:冶继民
报告平台:腾讯会议,ID:532-593-073
报告时间:2021年12月11日下午2:00-4:00
报告人简介:韩艺欣,南开大学统计与数据科学学院博士生,师从王兆军教授和邹长亮教授。于2019年赴美国佐治亚大学访学交流,合作导师为马平教授和钟文瑄教授。感兴趣的研究方向主要包括big data optimal subsampling; high-dimensional data inference; sufficient dimension reduction等。部分工作已在STATISTICA SINICA等国际高水平杂志发表。
报告摘要:Lack-of-fit testing is often essential in many statistical/machine learning applications. Despite the availability of large-scale data sets, the challenges associated with model checking when some resource budgets are limited are not yet well addressed. In this paper, we propose a design-adaptive testing procedure for checking a general model when only a limited number of data observations are available. We derive an optimal sampling strategy, called Structure-Adaptive-Sampling, to select a small subset from a large pool of data. With this subset, the proposed test possesses the asymptotically best power. Numerical results on both synthetic and real-world data confirm the effectiveness of the proposed method.