学术沙龙主题: Experimental design and Deterministic Sampling
报告人: 孙法省 东北师范大学 教授
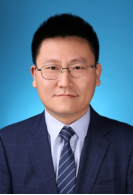
邀请人:李本崇
报告时间: 2021年10月31日(周日)上午9:30开始
报告地点:腾讯会议ID:479 935 513
报告人简介:孙法省,东北师范大学教授、博导,吉林省优秀教师,教育部“长江学者奖励计划”青年学者。博士毕业于南开大学概率论与数理统计专业,分别在加拿大西蒙弗雷泽大学统计与保险系、加州大学洛杉矶分校统计系做访问学者。主要从事计算机试验设计与数据分析、大数据抽样与统计推断等领域的研究。获教育部高校科学研究优秀成果奖(科学技术)自然科学奖二等奖,全国统计科学研究优秀成果奖、吉林省自然科学学术成果奖。已在统计学期刊 Annals of Statistics、 Journal of American Statistical Association、Biometrika 等期刊上发表论文20余篇,主持国家自然科学基金项目3项。
报告摘要: This paper introduces a new way to extract a set of representative points from a continuous distribution. These points are generated by minimizing the Kullback-Leibler divergence, which is an information-based measure of the disparity between two probability distributions. We refer these points as Kullback-Leibler points. Based on the link between the total variation and the Kullback-Leibler divergence, we prove that the empirical distribution of Kullback-Leibler points converges to the target distribution. Additionally, we illustrate that Kullback-Leibler points have advantages in simulations when compared with representative points generated by Monte Carlo or other deterministic sampling methods. In addition, to prevent the frequent evaluation of expensive distribution, an adaptive version of Kullback-Leibler points is proposed, which adaptively updates the representative points by learning about the complex or unknown distribution sequentially. Kullback-Leibler points can be used in the simulation of complex probability densities and the exploration and optimization of expensive black-box functions.