报告题目: Functional Regression on Manifold with Contamination
报 告 人: 姚方 博雅讲席教授/主任 北京大学统计科学中心
照 片:
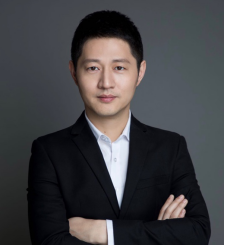
邀 请 人: 薄立军,冶继民
报告时间: 2020年9月9日(周三)10:00开始
报告地点:腾讯会议ID:932 719 167
报告人简介:北京大学博雅讲席教授,北大统计科学中心主任,国际数理统计学会(IMS)Fellow,美国统计学会(ASA)Fellow,现任IMS 理事会成员。2000年本科毕业于中国科技大学统计专业,2003获得加利福尼亚大学戴维斯分校统计学博士学位,曾任职于多伦多大学统计科学系终身教授。现担任Canadian Journal of Statistics的主编,至今担任9个国际统计学核心期刊编委,包括统计学顶级期刊Journal of the American Statistical Association和 Annals of Statistics。
报告摘要: We propose a new method for functional nonparametric regression with a predictor that resides on a finite-dimensional manifold but is only observable in an infinite-dimensional space. Contamination of the predictor due to discrete/noisy measurements is also accounted for. By using functional local linear manifold smoothing, the proposed estimator enjoys a polynomial rate of convergence that adapts to the intrinsic manifold dimension and the contamination level. This is in contrast to the logarithmic convergence rate in the literature of functional nonparametric regression. We also observe a phase transition phenomenon regarding the interplay of the manifold dimension and the contamination level. We demonstrate that the proposed method has favorable numerical performance relative to commonly used methods via simulated and real data examples.