威斯康星大学张春明教授系列学术报告1
报告题目:On simultaneous calibration of two-sample t-tests for high-dimension low-sample-size data
报 告 人:Chunming Zhang, Professor of Statistics, University of Wisconsin-Madison
照片:
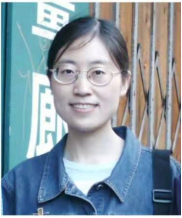
邀 请 人:冶 继 民 教 授
报告时间:2020年6月10号上午9:00:-10:30
报告平台:Zoom ID:865 6191 9897(密码2ArL1w)
报告人简介:张春明,美国威斯康星大学国际著名统计学教授,统计学四大顶级期刊之一Annals of Statistics的前副主编、Journal of the American Statistical Association的的现任副主编。在统计学四大顶级期刊发表论文10余篇,主要研究领域有:Statistical learning & data mining;Statistical methods with applications to imaging neuroinformatics and bioinformatics;Multiple testing; large-scale simultaneous inference and applications;Statistical methods in financial econometrics;Non- and semi-parametric estimation & inference;Functional & longitudinal data analysis.
报告摘要:Two-sample t-tests have been widely used in research practice and applications. This paper addresses new issues in simultaneously calibrating a diverging number m of two-sample t-statistics for simultaneous inference of significance in high-dimension low-sample size data. For the Gaussian calibration method, we demonstrate that (a) the simultaneous "general" two-sample t-statistics achieve the overall significance level, if log(m) increases at a strictly slower rate than (n_1+n_2)^{1/3} as n_1+n_2 diverges; (b) however, directly applying the same calibration method to simultaneous "pooled" two-sample t-statistics may substantially lose the overall level accuracy. The proposed "adaptively pooled" two-sample t-statistics overcome such incoherence, whereas operate as simply as but perform as well as the "general" two-sample t-statistics. (c) Moreover, we propose a "two-stage" t-test procedure to effectively alleviate the skewness effects commonly encountered from various two-sample t-statistics in practice, thus enhancing the calibration accuracy. Implications of these results are illustrated using simulation studies and real-data applications.