报告题目:MANY OBJECTIVE EVOLUTIONARY OPTIMIZATION AND KNEE-BASED MULTI-CRITERIA DECISION MAKING
报告人:Gary G. Yen 教授 美国俄克拉荷马州立大学
照片:
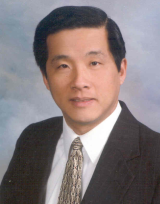
邀请人:高卫峰
报告时间:2019年7月26 15:00
报告地点:信远楼II205数统院报告厅
报告人简介:
Gary G. Yen教授于1992年在美国圣母大学获电气与计算机工程专业的博士学位。目前为美国俄克拉荷马州立大学电气与计算机工程学院的Regents讲席教授(Regents Professor)。Yen教授是国际智能控制、计算智能、多目标优化等领域著名专家学者,在智能控制、多目标优化、健康监测及其工业/国防应用做出了突出的工作。Gary在1994-1999年期间担任IEEE Transactions on Neural Networks和IEEE Control Systems Magazine的副主编,2000-2010年期间是IEEE Transactions on Control Systems Technology、 IEEE Transactions on Systems, Man and Cybernetics、 IFAC Journal on Automatica and Mechatronics的副主编。他目前是IEEE Transactions on Evolutionary Computation 和IEEE Transactions on Cybernetics的副主编。Gary于2004-2005年担任IEEE计算智能学会技术活动副主席,2006-2009年担任IEEE Computational Intelligence Magazine的创始主编。他曾在2010-2011年担任IEEE计算智能学会主席,并被选为2012-2014年IEEE杰出讲师。他于2009年获得了俄克拉荷马州立大学的Regents杰出研究奖,2011年获得了IEEE系统、人与控制论学会的Andrew P Sage最佳论文奖,2013年获得了IEEE计算智能学会的卓越服务奖,2014年获得了洛克希德马丁航空公司卓越教学奖。他是IEEE Fellow和IET Fellow。
报告摘要:
Evolutionary computation is a branch of studying biologically motivated computational paradigms which exert novel ideas and inspiration from natural evolution and adaptation. In recent years, one of the focuses has been advanced into the developing efficient and effective many-objective evolutionary algorithms. When encounter optimization problems with many objectives, nearly all designs perform poorly because of loss of selection pressure in fitness evaluation solely based upon Pareto optimality principle. In this talk, we will devote our attention to address three issues to complete the real-world applications at hand- visualization, performance measure and multi-criteria decision-making for the many-objective optimization. Visualization of population in a high-dimensional objective space throughout the evolution process presents an attractive feature that could be well exploited in designing many-objective evolutionary algorithms. A performance metric tailored specifically for many-objective optimization is critically needed, preventing various artifacts of existing performance metrics violating Pareto optimality principle. A minimum Manhattan distance (MMD) approach to multiple criteria decision making in many-objective optimization problems is considered. This procedure is equivalent to the knee selection described by a divide and conquer approach that involves iterations of pairwise comparisons. Given such a directive, knee-based evolutionary algorithms have also been exploited to address dynamic optimization, constraint optimization, robust optimization and their applications including automatic design of the deep neural networks. This talk will reveal some of most recent progresses been made in these research areas in making evolutionary computation a step closer to become a commercial-off-the-shelf solution.
主办单位:伟德国际BETVlCTOR